Azure Data Engineering Questions and Answers – 2023
1. What is Data Engineering?
Data Engineering is a field within the broader domain of data management that focuses on designing, building, and maintaining systems and infrastructure to support the collection, storage, processing, and analysis of large volumes of data. It plays a crucial role in the data lifecycle, ensuring that data is properly ingested, transformed, and made available for various data-driven applications and analytical processes.
The primary goal of data engineering is to create a robust and scalable data infrastructure that enables organizations to efficiently manage their data and extract meaningful insights from it. Data engineers are responsible for implementing data pipelines, integrating different data sources, and ensuring data quality and consistency. Here are some key aspects of data engineering:
1. Data Ingestion: Data engineers collect and ingest data from various sources, including databases, APIs, log files, sensors, and external systems. They design the data ingestion process to handle real-time or batch data streams, depending on the specific requirements of the organization.
2. Data Transformation: Raw data often needs to be processed and transformed into a usable format before it can be analyzed. Data engineers perform data transformations, such as data cleaning, enrichment, aggregation, and normalization, to make it suitable for analysis.
3. Data Storage: Data engineers choose appropriate storage solutions based on the volume, velocity, and variety of data. Common data storage technologies include relational databases, data warehouses, data lakes, and NoSQL databases.
4. Data Modeling: Data engineers create data models that define the structure and relationships of data within the organization. They may use data modeling techniques like entity-relationship diagrams or schema-on-read approaches to represent the data.
5. Data Governance and Security: Data engineers implement security measures and data governance policies to ensure data privacy, compliance with regulations, and data integrity.
6. ETL/ELT: ETL (Extract, Transform, Load) and ELT (Extract, Load, Transform) processes are crucial components of data engineering. ETL involves extracting data from various sources, transforming it, and then loading it into the target data storage. ELT involves loading raw data first and then performing transformations within the data storage system itself.
7. Big Data Technologies: In dealing with large-scale and high-velocity data, data engineers often work with big data technologies like Apache Hadoop, Apache Spark, and cloud-based data services.
Data engineering is fundamental for data-driven decision-making, business intelligence, machine learning, and various data analytics applications. It bridges the gap between raw data and meaningful insights, making it a crucial discipline in today’s data-driven world.
2. What is Azure Synapse analytics?
As of my last update in September 2021, Azure Synapse Analytics is an integrated analytics service provided by Microsoft as part of the Azure cloud platform. It combines big data and data warehousing capabilities into a single solution, enabling organizations to ingest, prepare, manage, and serve data for business intelligence, machine learning, and real-time analytics purposes. Azure Synapse Analytics was formerly known as Azure SQL Data Warehouse but was rebranded and expanded to offer more comprehensive analytics capabilities.
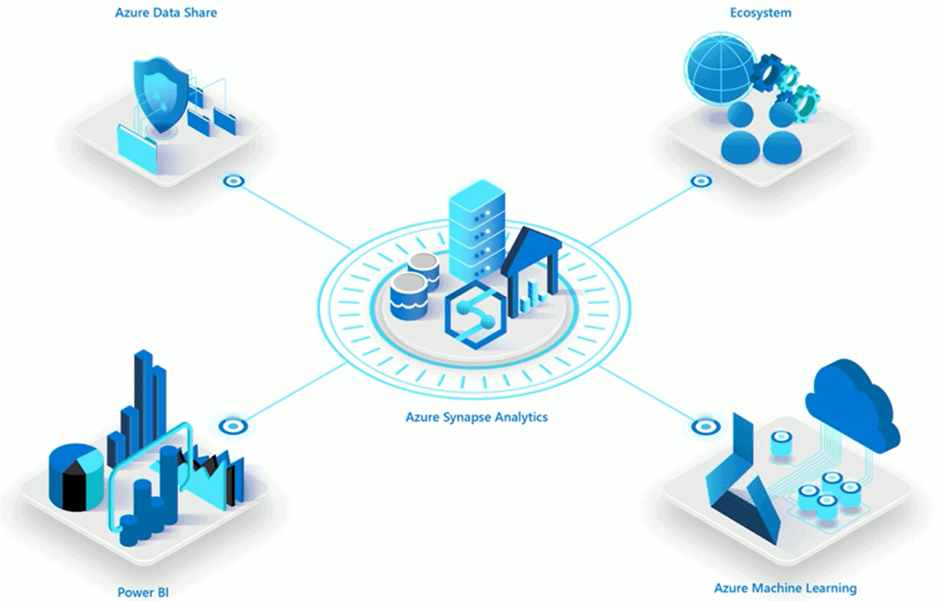
1. Unified Data Platform: It brings together data integration, data warehousing, big data analytics, and data exploration capabilities into a single unified platform, allowing users to work with diverse data sources seamlessly.
Key features of Azure Synapse Analytics include:
2. Data Integration: Azure Synapse Analytics provides built-in connectors and integration with various data sources, both on-premises and in the cloud, making it easier to ingest and process data from different systems.
3. Data Warehousing: It offers a distributed, scalable, and fully managed data warehousing solution to store and manage large volumes of structured and semi-structured data.
4. Big Data Analytics: The service allows users to perform big data analytics using Apache Spark, enabling data engineers and data scientists to analyze and process large datasets efficiently.
5. Real-time Analytics: Azure Synapse Analytics can be integrated with Azure Stream Analytics, enabling real-time data ingestion and analytics for streaming data scenarios.
6. Machine Learning Integration: It integrates with Azure Machine Learning, facilitating the deployment and operationalization of machine learning models on large datasets.
7. Serverless On-Demand Queries: Users can run serverless SQL queries on data residing in various locations, making it easier to analyze data without requiring a dedicated data warehouse.
8. Power BI Integration: Azure Synapse Analytics seamlessly integrates with Power BI, Microsoft’s business analytics service, enabling users to create interactive reports and dashboards.
9. Security and Governance: The service provides robust security features, including role-based access control, encryption, and data masking, ensuring data privacy and compliance with industry standards.
Overall, Azure Synapse Analytics is designed to provide an end-to-end analytics solution for modern data-driven businesses, allowing them to gain insights from large and diverse datasets efficiently and effectively. However, please note that Microsoft may have introduced new features or updates to Azure Synapse Analytics beyond my last update, so it’s always a good idea to refer to the official Azure documentation for the most current information.
3. Explain the data masking feature of Azure?
Data masking in Azure is a security feature that helps protect sensitive information by obfuscating or hiding sensitive data from unauthorized users or applications. It is an essential part of data security and compliance strategies, ensuring that only authorized individuals can access the actual sensitive data while others are provided with masked or scrambled representations.
Azure provides various tools and services to implement data masking, and one of the primary services used for this purpose is Azure SQL Database. Here’s an overview of how data masking works in Azure:
1. Sensitive Data Identification: The first step in data masking is to identify the sensitive data that needs protection. This includes personally identifiable information (PII), financial data, healthcare data, or any other information that could pose a risk if accessed by unauthorized users.
2. Data Masking Rules: Once sensitive data is identified, data masking rules are defined to specify how the data should be masked. These rules can vary based on the type of data and the level of security required. For example, data might be partially masked, fully masked, or substituted with random characters.
3. Data Masking Operations: When data masking is enabled, the data in the specified columns is automatically masked based on the defined rules. This process happens at the database level, ensuring that applications and users interacting with the database only see the masked data.
4. Access Control: Access control mechanisms, such as role-based access control (RBAC) and permissions, are used to ensure that only authorized users have access to the original (unmasked) data. Access to the masked data is typically provided to users who do not require access to the actual sensitive information.
5. Dynamic Data Masking: In some cases, dynamic data masking is used, which allows administrators to define masking rules dynamically at query runtime based on the user’s privileges. This means that different users may see different masked versions of the data based on their access rights.
Data masking is particularly beneficial in scenarios where developers, testers, or support personnel need access to production data for testing or troubleshooting purposes but should not be exposed to actual sensitive information. By using data masking, organizations can strike a balance between data privacy and the practical need to use realistic datasets for various purposes.
It’s important to note that while data masking provides an additional layer of security, it is not a substitute for robust access controls, encryption, or other security measures. A comprehensive data security strategy should include multiple layers of protection to safeguard sensitive information throughout its lifecycle.
4. Difference between Azure Synapse Analytics and Azure Data Lake Storage?
Feature | Azure Synapse Analytics | Azure Data Lake Storage |
Primary Purpose | Unified analytics service that combines big data and data warehousing capabilities. | Scalable and secure data lake storage for storing and managing large volumes of structured and unstructured data. |
Data Processing | Provides data warehousing, big data analytics (using Apache Spark), and real-time data processing. | Primarily focused on storing and managing data; data processing is typically performed using separate services or tools. |
Data Integration | Offers built-in connectors for integrating data from various sources for analysis. | Primarily focused on data storage, but data can be ingested and processed using other Azure services like Data Factory or Databricks. |
Query Language | Supports T-SQL (Transact-SQL) for querying structured data and Apache Spark SQL for big data analytics. | No built-in query language; data is typically accessed and processed through other Azure services or tools. |
Data Organization | Organizes data into dedicated SQL data pools and Apache Spark pools for different types of analytics. | Organizes data into hierarchical directories and folders, suitable for storing raw and processed data. |
Data Security | Provides robust security measures for data protection, access control, and auditing. | Offers granular access control through Azure Active Directory, and data can be encrypted at rest and in transit. |
Schema Management | Requires structured data with a predefined schema for SQL-based analytics. | Supports both structured and unstructured data, allowing schema-on-read for flexible data processing. |
Use Cases | Suitable for data warehousing, big data analytics, and real-time analytics scenarios. | Ideal for big data storage, data exploration, data archiving, and serving as a data source for various data processing workloads. |
Integration with Other Services | Integrates with other Azure services like Power BI, Azure Machine Learning, and Azure Stream Analytics. | Can be integrated with various Azure services like Data Factory, Databricks, and Azure HDInsight for data processing. |
5. Describe various windowing functions of Azure Stream Analytics?
Azure Stream Analytics provides several windowing functions that help you perform calculations on data streams within specific time or event intervals. These functions enable you to perform time-based aggregations, sliding window computations, and more. Here are some of the key windowing functions available in Azure Stream Analytics:
Tumbling Windows:
- Tumbling windows divide the data stream into fixed-size, non-overlapping time intervals.
- Each event belongs to one and only one tumbling window based on its timestamp.
- Useful for performing time-based aggregations over distinct time intervals.
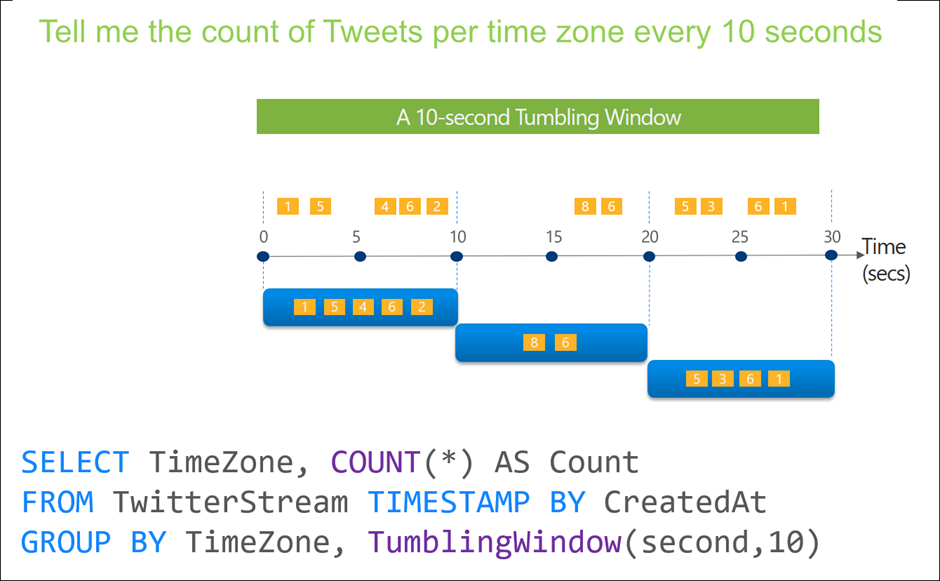

Hopping window
- Hopping windows are similar to tumbling windows but allow overlapping time intervals.
- You can specify the hop size and window size, determining how much overlap exists between adjacent windows.
- Useful for calculating aggregates over sliding time intervals.


Sliding window
- Sliding windows divide the data stream into fixed-size, overlapping time intervals.
- Unlike hopping windows, sliding windows always have an overlap between adjacent windows.
- You can specify the window size and the slide size.
- Useful for analyzing recent data while considering historical context.

With the following input data (illustrated above):

Session Windows:
- Session windows group events that are close together in time based on a specified gap duration.
- The gap duration is the maximum time interval between events that belong to the same session window.
- Useful for detecting and analyzing bursts or periods of activity in data streams.

With the following input data (illustrated above):

Snapshot window
Snapshot windows group events that have the same timestamp. Unlike other windowing types, which require a specific window function (such as SessionWindow()), you can apply a snapshot window by adding System.Timestamp() to the GROUP BY clause.
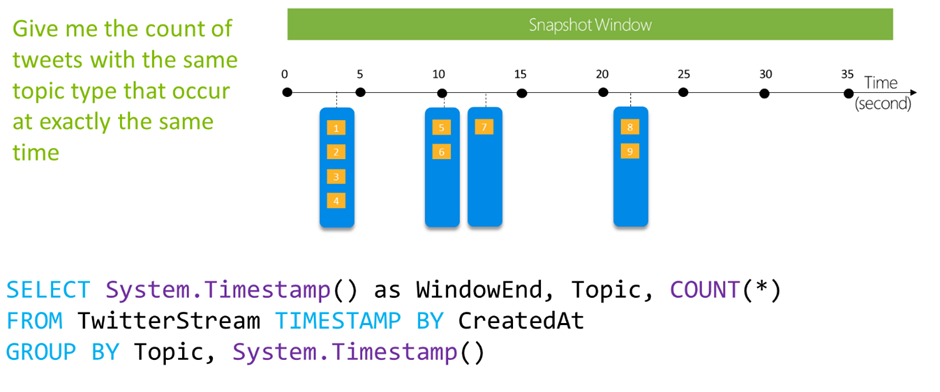

6. What are the different storage types in Azure?
The following are the various advantages of the Java collection framework:
Storage Types | Operations |
Files | Azure Files is an organized way of storing data on the cloud. The main advantage of using Azure Files over Azure Blobs is that Azure Files allows for organizing the data in a folder structure. Also, Azure Files is SMB (Server Message Block) protocol compliant, i.e., and can be used as a file share. |
Blobs | Blob stands for a large binary object. This storage solution supports all kinds of files, including text files, videos, images, documents, binary data, etc. |
Queues | Azure Queue is a cloud-based messaging store for establishing and brokering communication between various applications and components. |
Disks | The Azure disk is used as a storage solution for Azure VMs (Virtual Machines) |
Tables | Tables are NoSQL storage structures for storing structured data that does not meet the standard RDBMS (relational database schema). |

7. What are the different security options available in the Azure SQL database?
Azure SQL Database provides a range of security options to ensure the confidentiality, integrity, and availability of your data. These options are designed to protect your database from unauthorized access, data breaches, and other security threats. Here are some of the key security features available in Azure SQL Database:
1. Firewall Rules:
- Azure SQL Database allows you to configure firewall rules to control which IP addresses or IP address ranges can access your database.
- By default, all access from outside Azure’s datacenters is blocked until you define the necessary firewall rules.
2. Authentication:
- Azure SQL Database supports two types of authentications: SQL Authentication and Azure Active Directory Authentication.
- SQL Authentication uses usernames and passwords to authenticate users, while Azure Active Directory Authentication enables users to sign in with their Azure AD credentials.
3. Transparent Data Encryption (TDE):
- TDE automatically encrypts data at rest, providing an additional layer of security for your database.
- The data and log files are encrypted using a database encryption key, which is further protected by a service-managed certificate.
4. Always Encrypted:
- Always Encrypted is a feature that allows you to encrypt sensitive data in the database while keeping the encryption keys within your application.
- This ensures that even database administrators cannot access the plaintext data.
5. Auditing and Threat Detection:
- Azure SQL Database offers built-in auditing that allows you to track database events and store audit logs in Azure Storage.
- Threat Detection provides continuous monitoring and anomaly detection to identify potential security threats and suspicious activities.
6. Row-Level Security (RLS):
- RLS enables you to control access to rows in a database table based on the characteristics of the user executing a query.
- This feature is useful for implementing fine-grained access controls on a per-row basis.
7. Virtual Network Service Endpoints:
- With Virtual Network Service Endpoints, you can extend your virtual network’s private IP address space and route traffic securely to Azure SQL Database over the Azure backbone network.
- This helps to ensure that your database can only be accessed from specific virtual networks, improving network security.
8. Data Masking:
- Data Masking enables you to obfuscate sensitive data in query results, making it possible to share the data with non-privileged users without revealing the actual values.
9. Threat Protection:
- Azure SQL Database’s Threat Protection feature helps identify and mitigate potential database vulnerabilities and security issues.
10. Advanced Data Security (ADS):
- Advanced Data Security is a unified package that includes features like Vulnerability Assessment, Data Discovery & Classification, and SQL Injection Protection to enhance the overall security of your database.
By leveraging these security options, you can ensure that your Azure SQL Database remains protected against various security threats and maintain the confidentiality and integrity of your data. It is essential to configure and monitor these security features based on your specific requirements and compliance standards.
8. How data security is implemented in Azure Data Lake Storage(ADLS) Gen2?
Azure Data Lake Storage Gen2 (ADLS Gen2) provides robust data security features to protect data at rest and in transit. It builds on the security features of Azure Blob Storage and adds hierarchical namespace support, enabling it to function as both an object store and a file system. Here’s how data security is implemented in Azure Data Lake Storage Gen2:
1. Role-Based Access Control (RBAC):
- ADLS Gen2 leverages Azure RBAC to control access to resources at the Azure subscription and resource group levels.
- You can assign roles such as Storage Account Contributor, Storage Account Owner, or Custom roles with specific permissions to users, groups, or applications.
2. POSIX Access Control Lists (ACLs):
- ADLS Gen2 supports POSIX-like ACLs, allowing you to grant fine-grained access control to individual files and directories within the data lake.
- This enables you to define access permissions for specific users or groups, controlling read, write, and execute operations.
3. Shared Access Signatures (SAS):
- SAS tokens provide limited and time-bound access to specific resources in ADLS Gen2.
- You can generate SAS tokens with custom permissions and time constraints, which are useful for granting temporary access to external entities without exposing storage account keys.
4. Data Encryption:
- Data at rest is automatically encrypted using Microsoft-managed keys (SSE, Server-Side Encryption).
- Optionally, you can bring your encryption keys using customer-managed keys (CMEK) for an added layer of control.
5. Azure Private Link:
- ADLS Gen2 can be integrated with Azure Private Link, which allows you to access the service over a private, dedicated network connection (Azure Virtual Network).
- This helps to prevent data exposure to the public internet and enhances network security.
6. Firewall and Virtual Network Service Endpoints:
- ADLS Gen2 allows you to configure firewall rules to control which IP addresses or IP address ranges can access the data lake.
- Virtual Network Service Endpoints enable secure access to the data lake from within an Azure Virtual Network.
7. Data Classification and Sensitivity Labels:
- ADLS Gen2 supports Azure Data Classification and Sensitivity Labels, allowing you to classify and label data based on its sensitivity level.
- These labels can be used to enforce policies, auditing, and access control based on data classification.
8. Auditing and Monitoring:
- ADLS Gen2 offers auditing capabilities that allow you to track access to the data lake and log events for compliance and security monitoring.
- You can integrate ADLS Gen2 with Azure Monitor to get insights into the health and performance of the service.
By combining these security features, Azure Data Lake Storage Gen2 ensures that your data is protected from unauthorized access, tampering, and data breaches. It is crucial to configure these security options based on your specific data security requirements and compliance standards to safeguard your data effectively.
9. What are the various data flow partition schemes available in Azure?
Partition Scheme | Explanation | Usage |
Round Robin | It is the most straightforward partition scheme which spreads data evenly across partitions. | No good key candidates were available in the data. |
Hash | Hash of columns creates uniform partitions such that rows with similar values fall in the same partition. | It is used to check for partition skew. |
Dynamic Range | Spark dynamics range based on the provided columns or expression. | Select the column that will be used for partitioning. |
Fixed Range | A fixed range of values based on the user-created expression for disturbing data across partitions. | A good understanding of data is required to avoid partition skew. |
Key | Partition for each unique value in the selected column. | Good understanding of data cardinality is required. |
10. Why is the Azure data factory needed?
Azure Data Factory is a cloud-based data integration service provided by Microsoft Azure. It is designed to address the challenges of data movement and data orchestration in modern data-centric environments. The primary reasons why Azure Data Factory is needed are as follows:
1. Data Integration and Orchestration: In today’s data landscape, organizations often deal with data spread across various sources and formats, both on-premises and in the cloud. Azure Data Factory enables seamless integration and orchestration of data from diverse sources, making it easier to collect, transform, and move data between systems.
2. ETL (Extract, Transform, Load) Workflows: ETL processes are fundamental in data warehousing and analytics. Azure Data Factory allows you to create complex data workflows, where you can extract data from different sources, apply transformations, and load it into the target destination efficiently.
3. Serverless and Scalable: Azure Data Factory is a serverless service, meaning you don’t need to manage the underlying infrastructure. It automatically scales up or down based on demand, ensuring that you can process data of any volume without worrying about infrastructure limitations.
4. Integration with Azure Services: As part of the Azure ecosystem, Data Factory seamlessly integrates with other Azure services like Azure Blob Storage, Azure SQL Database, Azure Data Lake, Azure Databricks, etc. This integration enhances data processing capabilities and enables users to take advantage of Azure’s broader suite of analytics and storage solutions.
5. Data Transformation and Data Flow: Azure Data Factory provides data wrangling capabilities through data flows, allowing users to build data transformation logic visually. This simplifies the process of data cleansing, enrichment, and preparation for analytics or reporting.
6. Monitoring and Management: Azure Data Factory comes with built-in monitoring and management tools that allow you to track the performance of your data pipelines, troubleshoot issues, and set up alerts for critical events.
7. Hybrid Data Movement: For organizations with a hybrid cloud strategy or data stored on-premises, Azure Data Factory offers connectivity to on-premises data sources using a secure data gateway. This enables smooth integration of cloud and on-premises data.
8. Time Efficiency: Data Factory enables you to automate data pipelines and schedule data movements and transformations, reducing manual intervention and saving time in the data integration process.
Overall, Azure Data Factory plays a crucial role in simplifying data integration, enabling organizations to gain insights from their data, and facilitating the development of robust data-driven solutions in the Azure cloud environment.
11. What is Azure Data Factory?
Azure Data Factory is a cloud-based integration service offered by Microsoft that lets you create data-driven workflows for orchestrating and automating data movement and data transformation overcloud. Data Factory services also offer to create and running data pipelines that move and transform data and then run the pipeline on a specified schedule.
12. What is Integration Runtime?
Integration runtime is nothing but a compute structure used by Azure Data Factory to give integration capabilities across different network environments.
Types of Integration Runtimes:
- Azure Integration Runtime – It can copy data between cloud data stores and dispatch the activity to a variety of computing services such as SQL Server, Azure HDInsight
- Self Hosted Integration Runtime – It’s software with basically the same code as Azure Integration runtime, but it’s installed on on- premises systems or virtual machines over virtual networks.
- Azure SSIS Integration Runtime – It helps to execute SSIS packages in a managed environment. So when we lift and shift the SSIS packages to the data factory, we use Azure SSIS Integration Runtime.
13. What are the different components used in Azure Data Factory?
Azure Data Factory consists of several numbers of components. Some components are as follows:
- Pipeline: The pipeline is the logical container of the activities.
- Activity: It specifies the execution step in the Data Factory pipeline, which is substantially used for data ingestion and metamorphosis.
- Dataset: A dataset specifies the pointer to the data used in the pipeline conditioning.
- Mapping Data Flow: It specifies the data transformation UI logic.
- Linked Service: It specifies the descriptive connection string for the data sources used in the channel conditioning. Let‘s say we’ve an SQL server, so we need a connecting string connected to an external device, and we will mention the source and the destination for it.
- Trigger: It specifies the time when the pipeline will be executed.
- Control flow: It’s used to control the execution flow of the pipeline activities
14. What is the key difference between the Dataset and Linked Service in Azure Data Factory?
Dataset specifies a source to the data store described by the linked service. When we put data to the dataset from a SQL Server instance, the dataset indicates the table’s name that contains the target data or the query that returns data from dissimilar tables.
Linked service specifies a definition of the connection string used to connect to the data stores. For illustration, when we put data in a linked service from a SQL Server instance, the linked service contains the name for the SQL Server instance and the credentials used to connect to that case.
15. What is the difference between Azure Data Lake and Azure Data Warehouse?

Azure Data Lake | Data Warehouse |
Data Lake is a capable way of storing any type, size, and shape of data. | Data Warehouse acts as a repository for already filtered data from a specific resource. |
It is mainly used by Data Scientists. | It is more frequently used by Business Professionals. |
It is highly accessible with quicker updates. | It becomes a pretty rigid and costly task to make changes in Data Warehouse. |
It defines the schema after when the data is stored successfully. | Datawarehouse defines the schema before storing the data. |
It uses ELT (Extract, Load and Transform) process. | It uses ETL (Extract, Transform and Load) process. |
It is an ideal platform for doing in-depth analysis. | It is the best platform for operational users. |
16. Difference between Data Lake Storage and Blob Storage.
Data Lake Storage | Blob Storage |
It is an optimized storage solution for big data analytics workloads. | Blob Storage is general-purpose storage for a wide variety of scenarios. It can also do Big Data Analytics. |
It follows a hierarchical file system. | It follows an object store with a flat namespace. |
In Data Lake Storage, data is stored as files inside folders. | Blob storage lets you create a storage account. Storage account has containers that store the data. |
It can be used to store Batch, interactive, stream analytics, and machine learning data. | We can use it to store text files, binary data, media storage for streaming and general purpose data. |
16. What are the steps to create an ETL process in Azure Data Factory?
The ETL (Extract, Transform, Load) process follows four main steps:
i) Connect and Collect: Connect to the data source/s and move data to local and crowdsource data storage.
ii) Data transformation using computing services such as HDInsight, Hadoop, Spark, etc.
iii) Publish: To load data into Azure data lake storage, Azure SQL data warehouse, Azure SQL databases, Azure Cosmos DB, etc.
iv)Monitor: Azure Data Factory has built-in support for pipeline monitoring via Azure Monitor, API, PowerShell, Azure Monitor logs, and health panels on the Azure portal.
17. What are the key differences between the Mapping data flow and Wrangling data flow transformation activities in Azure Data Factory?
In Azure Data Factory, the main dissimilarity between the Mapping data flow and the Wrangling data flow transformation activities is as follows
The Mapping data flow activity is a visually allowed data transformation activity that facilitates users to plan graphical data transformation logic. It does not need the users to be expert developers. It’s executed as an activity within the ADF pipeline on an ADF completely managed scaled-out Spark cluster.
On the other hand, the Wrangling data flow activity is a code–free data preparation activity. It’s integrated with Power Query Online to make the Power Query M functions available for data wrangling using spark execution.
18. Can we pass parameters to a pipeline run?
Yes definitely, we can very easily pass parameters to a pipeline run. Pipeline runs are the first-class, top-level concepts in Azure Data Factory. We can define parameters at the pipeline level, and then we can pass the arguments to run a pipeline.
You can also define default values for the parameters in the pipeline.
19. Can an activity in a pipeline consume arguments that are passed to a pipeline run?
Each activity within the pipeline can consume the parameter value that’s passed to the pipeline and run with the @parameter construct.
Parameters are a first-class, top-level concept in Data Factory. We can define parameters at the pipeline level and pass arguments as you execute the pipeline run on demand or using a trigger.
20. Can an activity output property be consumed in another activity?
An activity output can be consumed in a subsequent activity with the @activity construct.
21. How do I gracefully handle null values in an activity output?
You can use the @coalesce construct in the expressions to handle the null values gracefully.
23. What has changed from private preview to limited public preview in regard to data flows?
There are a couple of things which have been changed mentioned below:
- You are no longer required to bring your own Azure Databricks Clusters.
- Data Factory will manage cluster creation and tear down process.
- We can still use Data Lake Storage Gen 2 and Blob Storage to store those files. You can use the appropriate linked services. You can also use the appropriate linked services for those of the storage engines.
- Blob data sets and Azure Data Lake storage gen 2 are separated into delimited text and Apache Parquet datasets.
24. What is Azure SSIS Integration Runtime?
Azure SSIS Integration is a fully managed cluster of virtual machines that are hosted in Azure and dedicated to run SSIS packages in the data factory. We can easily scale up the SSIS nodes by configuring the node size or scaled out by configuring the number of nodes on the Virtual Machine’s cluster.
We must create an SSIS integration runtime and an SSISDB catalog hosted in the Azure SQL server database or Azure SQL-managed instance before executing an SSIS package
25. An Azure Data Factory Pipeline can be executed using three methods. Mention these methods.
Methods to execute Azure Data Factory Pipeline:
- Debug Mode
- Manual execution using trigger now
- Adding schedule, tumbling window/event trigger
26. Can we monitor and manage Azure Data Factory Pipelines?
Yes, we can monitor and manage ADF Pipelines using the following steps:
- Click on the monitor and manage on the data factory tab.
- Click on the resource manager.
- Here, you will find- pipelines, datasets, and linked services in a tree format.
27. What are the steps involved in the ETL process?
ETL (Extract, Transform, Load) process follows four main steps:
- Connect and Collect – helps in moving the data on-premises and cloud source data stores
- Transform – lets users collect the data by using compute services such as HDInsight Hadoop, Spark etc.
- Publish – Helps in loading the data into Azure data warehouse, Azure SQL database, and Azure Cosmos DB etc
- Monitor – It helps support the pipeline monitoring via Azure Monitor, API and PowerShell, Log Analytics, and health panels on the Azure Portal.
28. What are the different ways to execute pipelines in Azure Data Factory?
There are three ways in which we can execute a pipeline in Data Factory:
- Debug mode can be helpful when trying out pipeline code and acts as a tool to test and troubleshoot our code.
- Manual Execution is what we do by clicking on the ‘Trigger now’ option in a pipeline. This is useful if you want to run your pipelines on an ad-hoc basis.
- We can schedule our pipelines at predefined times and intervals via a Trigger. As we will see later in this article, there are three types of triggers available in Data Factory.
29. What is the purpose of Linked services in Azure Data Factory?
Linked services are used majorly for two purposes in Data Factory:
- For a Data Store representation, i.e., any storage system like Azure Blob storage account, a file share, or an Oracle DB/ SQL Server instance.
- For Compute representation, i.e., the underlying VM will execute the activity defined in the pipeline.
30. Can you Elaborate more on Data Factory Integration Runtime?
The Integration Runtime, or IR, is the compute infrastructure for Azure Data Factory pipelines. It is the bridge between activities and linked services. The linked Service or Activity references it and provides the computing environment where the activity is run directly or dispatched. This allows the activity to be performed in the closest region to the target data stores or computing Services.
The following diagram shows the location settings for Data Factory and its integration runtimes:

Azure Data Factory supports three types of integration runtime, and one should choose based on their data integration capabilities and network environment requirements.
- Azure Integration Runtime: To copy data between cloud data stores and send activity to various computing services such as SQL Server, Azure HDInsight, etc.
- Self-Hosted Integration Runtime: Used for running copy activity between cloud data stores and data stores in private networks. Self-hosted integration runtime is software with the same code as the Azure Integration Runtime but installed on your local system or machine over a virtual network.
- Azure SSIS Integration Runtime: You can run SSIS packages in a managed environment. So, when we lift and shift SSIS packages to the data factory, we use Azure SSIS Integration Runtime.
31. What are ARM Templates in Azure Data Factory? What are they used for?
An ARM template is a JSON (JavaScript Object Notation) file that defines the infrastructure and configuration for the data factory pipeline, including pipeline activities, linked services, datasets, etc. The template will contain essentially the same code as our pipeline.
ARM templates are helpful when we want to migrate our pipeline code to higher environments, say Production or Staging from Development, after we are convinced that the code is working correctly.
32. What are the two types of compute environments supported by Data Factory to execute the transform activities?
Below are the types of computing environments that Data Factory supports for executing transformation activities: –
i) On-Demand Computing Environment: This is a fully managed environment provided by ADF. This type of calculation creates a cluster to perform the transformation activity and automatically deletes it when the activity is complete.
ii) Bring Your Environment: In this environment, you can use ADF to manage your computing environment if you already have the infrastructure for on-premises services.
33. If you want to use the output by executing a query, which activity shall you use?
Look-up activity can return the result of executing a query or stored procedure.
The output can be a singleton value or an array of attributes, which can be consumed in subsequent copy data activity, or any transformation or control flow activity like ForEach activity.
34. What are some useful constructs available in Data Factory?
- parameter: Each activity within the pipeline can consume the parameter value passed to the pipeline and run with the @parameter construct.
- coalesce: We can use the @coalesce construct in the expressions to handle null values gracefully.
- activity: An activity output can be consumed in a subsequent activity with the @activity construct.
35. Can we push code and have CI/CD (Continuous Integration and Continuous Delivery) in ADF?
Data Factory fully supports CI/CD of your data pipelines using Azure DevOps and GitHub. This allows you to develop and deliver your ETL processes incrementally before publishing the finished product. After the raw data has been refined into a business-ready consumable form, load the data into Azure Data Warehouse or Azure SQL Azure Data Lake, Azure Cosmos DB, or whichever analytics engine your business uses can point to from their business intelligence tools.
36. What do you mean by variables in the Azure Data Factory?
Variables in the Azure Data Factory pipeline provide the functionality to hold the values. They are used for a similar reason as we use variables in any programming language and are available inside the pipeline.
Set variables and append variables are two activities used for setting or manipulating the values of the variables. There are two types of variables in a data factory: –
i) System variables: These are fixed variables from the Azure pipeline. For example, pipeline name, pipeline id, trigger name, etc. You need these to get the system information required in your use case.
ii) User variable: A user variable is declared manually in your code based on your pipeline logic.
37. What are the different activities you have used in Azure Data Factory?
Here you can share some of the significant activities if you have used them in your career, whether your work or college project. Here are a few of the most used activities :
- Copy Data Activity to copy the data between datasets.
- ForEach Activity for looping.
- Get Metadata Activity that can provide metadata about any data source.
- Set Variable Activity to define and initiate variables within pipelines.
- Lookup Activity to do a lookup to get some values from a table/file.
- Wait Activity to wait for a specified amount of time before/in between the pipeline run.
- Validation Activity will validate the presence of files within the dataset.
- Web Activity to call a custom REST endpoint from an ADF pipeline.
38. How can I schedule a pipeline?
You can use the time window or scheduler trigger to schedule a pipeline. The trigger uses a wall-clock calendar schedule, which can schedule pipelines periodically or in calendar-based recurrent patterns (for example, on Mondays at 6:00 PM and Thursdays at 9:00 PM).
Currently, the service supports three types of triggers:
- Tumbling window trigger: A trigger that operates on a periodic interval while retaining a state.
- Schedule Trigger: A trigger that invokes a pipeline on a wall-clock schedule.
- Event-Based Trigger: A trigger that responds to an event. e.g., a file getting placed inside a blob.
Pipelines and triggers have a many-to-many relationship (except for the tumbling window trigger). Multiple triggers can kick off a single pipeline, or a single trigger can kick off numerous pipelines.

39. How can you access data using the other 90 dataset types in Data Factory?
The mapping data flow feature allows Azure SQL Database, Azure Synapse Analytics, delimited text files from Azure storage account or Azure Data Lake Storage Gen2, and Parquet files from blob storage or Data Lake Storage Gen2 natively for source and sink data source. Use the Copy activity to stage data from any other connectors and then execute a Data Flow activity to transform data after it’s been staged.
40. Can a value be calculated for a new column from the existing column from mapping in ADF?
We can derive transformations in the mapping data flow to generate a new column based on our desired logic. We can create a new derived column or update an existing one when developing a derived one. Enter the name of the column you’re making in the Column textbox.
You can use the column dropdown to override an existing column in your schema. Click the Enter expression textbox to start creating the derived column’s expression. You can input or use the expression builder to build your logic.
41. How is the lookup activity useful in the Azure Data Factory?
In the ADF pipeline, the Lookup activity is commonly used for configuration lookup purposes, and the source dataset is available. Moreover, it retrieves the data from the source dataset and then sends it as the activity output. Generally, the output of the lookup activity is further used in the pipeline for making decisions or presenting any configuration as a result.
Simply put, lookup activity is used for data fetching in the ADF pipeline. The way you would use it entirely relies on your pipeline logic. Obtaining only the first row is possible, or you can retrieve the complete rows depending on your dataset or query.
42. Elaborate more on the Get Metadata activity in Azure Data Factory.
The Get Metadata activity is used to retrieve the metadata of any data in the Azure Data Factory or a Synapse pipeline. We can use the output from the Get Metadata activity in conditional expressions to perform validation or consume the metadata in subsequent activities.
It takes a dataset as input and returns metadata information as output. Currently, the following connectors and the corresponding retrievable metadata are supported. The maximum size of returned metadata is 4 MB.
Please refer to the snapshot below for supported metadata which can be retrieved using the Get Metadata activity.

43. What does it mean by the breakpoint in the ADF pipeline?
To understand better, for example, you are using three activities in the pipeline, and now you want to debug up to the second activity only. You can do this by placing the breakpoint at the second activity. To add a breakpoint, click the circle present at the top of the activity.
44. Can you share any difficulties you faced while getting data from on-premises to Azure cloud using Data Factory?
One of the significant challenges we face while migrating from on-prem to the cloud is throughput and speed. When we try to copy the data using Copy activity from on-prem, the process rate could be faster, and hence we need to get the desired throughput.
There are some configuration options for a copy activity, which can help in tuning this process and can give desired results.
i) We should use the compression option to get the data in a compressed mode while loading from on-prem servers, which is then de-compressed while writing on the cloud storage.
ii) Staging area should be the first destination of our data after we have enabled the compression. The copy activity can decompress before writing it to the final cloud storage buckets.
iii) Degree of Copy Parallelism is another option to help improve the migration process. This is identical to having multiple threads processing data and can speed up the data copy process.
There is no right fit-for-all here, so we must try different numbers like 8, 16, or 32 to see which performs well.
iv) Data Integration Unit is loosely the number of CPUs used, and increasing it may improve the performance of the copy process.
45. How to copy multiple sheet data from an Excel file?
When using an Excel connector within a data factory, we must provide a sheet name from which we must load data. This approach is nuanced when we have to deal with a single or a handful of sheets of data, but when we have lots of sheets (say 10+), this may become a tedious task as we have to change the hard-coded sheet name every time!
However, we can use a data factory binary data format connector for this and point it to the Excel file and need not provide the sheet name/s. We’ll be able to use copy activity to copy the data from all the sheets present in the file.
46. Is it possible to have nested looping in Azure Data Factory?
There is no direct support for nested looping in the data factory for any looping activity (for each / until). However, we can use one for each/until loop activity which will contain an execute pipeline activity that can have a loop activity. This way, when we call the looping activity, it will indirectly call another loop activity, and we’ll be able to achieve nested looping.
47. How to copy multiple tables from one datastore to another datastore?
An efficient approach to complete this task would be:
- Maintain a lookup table/ file containing the list of tables and their source, which needs to be copied.
- Then, we can use the lookup activity and each loop activity to scan through the list.
- Inside the for each loop activity, we can use a copy activity or a mapping dataflow to copy multiple tables to the destination datastore.
48. What are some performance-tuning techniques for Mapping Data Flow activity?
We could consider the below set of parameters for tuning the performance of a Mapping Data Flow activity we have in a pipeline.
i) We should leverage partitioning in the source, sink, or transformation whenever possible. Microsoft, however, recommends using the default partition (size 128 MB) selected by the Data Factory as it intelligently chooses one based on our pipeline configuration.
Still, one should try out different partitions and see if they can have improved performance.
ii) We should not use a data flow activity for each loop activity. Instead, we have multiple files similar in structure and processing needs. In that case, we should use a wildcard path inside the data flow activity, enabling the processing of all the files within a folder.
iii) The recommended file format to use is ‘. parquet’. The reason being the pipeline will execute by spinning up spark clusters, and Parquet is the native file format for Apache spark thus, it will generally give good performance.
iv) Multiple logging modes are available: Basic, Verbose, and None.
We should only use verbose mode if essential, as it will log all the details about each operation the activity performs. e.g., It will log all the details of the operations performed for all our partitions. This one is useful when troubleshooting issues with the data flow.
The basic mode will give out all the necessary basic details in the log, so try to use this one whenever possible.
v) Try to break down a complex data flow activity into multiple data flow activities. Let’s say we have several transformations between source and sink, and by adding more, we think the design has become complex. In this case, try to have it in multiple such activities, which will give two advantages:
- All activities will run on separate spark clusters, decreasing the run time for the whole task.
- The whole pipeline will be easy to understand and maintain in the future.
49. What are some of the limitations of ADF?
Azure Data Factory provides great functionalities for data movement and transformations. However, there are some limitations as well.
i) We can’t have nested looping activities in the data factory, and we must use some workaround if we have that sort of structure in our pipeline. All the looping activities come under this: If, Foreach, switch, and until activities.
ii) The lookup activity can retrieve only 5000 rows at a time and not more than that. Again, we need to use some other loop activity along with SQL with the limit to achieve this sort of structure in the pipeline.
iii) We can have 40 activities in a single pipeline, including inner activity, containers, etc. To overcome this, we should modularize the pipelines regarding the number of datasets, activities, etc.
50. How are all the components of Azure Data Factory combined to complete an ADF task?
The below diagram depicts how all these components can be clubbed together to fulfill Azure Data Factory ADF tasks.

51. How do you send email notifications on pipeline failure?
There are multiple ways to do this:
- Using Logic Apps with Web/Webhook activity.
Configure a logic app that, upon getting an HTTP request, can send an email to the required set of people for failure. In the pipeline, configure the failure option to hit the URL generated by the logic app. - Using Alerts and Metrics from pipeline options.
We can set up this from the pipeline itself, where we get numerous options for email on any activity failure within the pipeline.
52. Imagine you need to process streaming data in real time and store the results in an Azure Cosmos DB database. How would you design a pipeline in Azure Data Factory to efficiently handle the continuous data stream and ensure it is correctly stored and indexed in the destination database?
Here are the steps to design a pipeline in Azure Data Factory to efficiently handle streaming data and store it in an Azure Cosmos DB database.
- Set up an Azure Event Hub or Azure IoT Hub as the data source to receive the streaming data.
- Use Azure Stream Analytics to process and transform the data in real time using Stream Analytics queries.
- Write the transformed data to a Cosmos DB collection as an output of the Stream Analytics job.
- Optimize query performance by configuring appropriate indexing policies for the Cosmos DB collection.
- Monitor the pipeline for issues using Azure Data Factory’s monitoring and diagnostic features, such as alerts and logs.
54. How can one combine or merge several rows into one row in ADF? Can you explain the process?
In Azure Data Factory (ADF), you can merge or combine several rows into a single row using the “Aggregate” transformation.
55. How do you copy data as per file size in ADF?
You can copy data based on file size by using the “FileFilter” property in the Azure Data Factory. This property allows you to specify a file pattern to filter the files based on size.
Here are the steps you can follow to copy data based on the file size:
- Create a dataset for the source and destination data stores.
- Now, set the “FileFilter” property to filter the files based on their size in the source dataset.
- In the copy activity, select the source and destination datasets and configure the copy behavior per your requirement.
- Run the pipeline to copy the data based on the file size filter.
56. How can you insert folder name and file count from blob into SQL table?
You can follow these steps to insert a folder name and file count from blob into the SQL table:
- Create an ADF pipeline with a “Get Metadata” activity to retrieve the folder and file details from the blob storage.
- Add a “ForEach” activity to loop through each folder in the blob storage.
- Inside the “ForEach” activity, add a “Get Metadata” activity to retrieve the file count for each folder.
- Add a “Copy Data” activity to insert the folder name and file count into the SQL table.
- Configure the “Copy Data” activity to use the folder name and file count as source data and insert them into the appropriate columns in the SQL table.
- Run the ADF pipeline to insert the folder name and file count into the SQL table.
57. What are the various types of loops in ADF?
Loops in Azure Data Factory are used to iterate over a collection of items to perform a specific action repeatedly. There are three major types of loops in Azure Data Factory:
- For Each Loop: This loop is used to iterate over a collection of items and perform a specific action for each item in the collection. For example, if you have a list of files in a folder and want to copy each file to another location, you can use a For Each Loop to iterate over the list of files and copy each file to the target location.
- Until Loop: This loop repeats a set of activities until a specific condition is met. For example, you could use an Until Loop to keep retrying an operation until it succeeds or until a certain number of attempts have been made.
- While Loop: This loop repeats a specific action while a condition is true. For example, if you want to keep processing data until a specific condition is met, you can use a While Loop to repeat the processing until the condition is no longer true.
58. What is Data factory parameterization?
Data factory allows for parameterization of pipelines via three elements:
- Parameters
- Variables
- Expression
Parameter:
Parameters are simply input values for operations in data factory. Each action has a set of predefined parameters that need to be supplied.
Additionally, some blocks like pipeline and datasets allow you to define custom parameters.
Variables:
Variable, set values inside the pipelines using Set variable function. Variables are temporary values that are used within pipeline and workflow to control execution of the workflow.
Variable can be modified through expression using Set variable action during the execution of the work flow.
Variables support 3 data types: string, bool and array. We refer these user variable as below @variables(‘variableName’)
Expression:
Expression is a JSON based formula, which allow for modification of variable or any parameters for pipeline, action or connection in data factory.
Typical scenario:
Most common scenarios that mandate parameterization are:
- Dynamic input file name coming from external service
- Dynamic output table name
- Appending date to output
- Changing connection parameter name like database name
- Conditional programming
- And many more…
59. What are the different Slowly changing dimension types?
Star schema design theory refers to common SCD types. The most common are Type 1 and Type 2. In practice a dimension table may support a combination of history tracking methods, including Type 3 and Type 6.
Type 1 SCD:
A Type 1 SCD always reflects the latest values, and when changes in source data are detected, the dimension table data is overwritten. This design approach is common for columns that store supplementary values, like the email address or phone number of a customer. When a customer email address or phone number changes, the dimension table updates the customer row with the new values. It’s as if the customer always had this contact information. The key field, such as CustomerID, would stay the same so the records in the fact table automatically link to the updated customer record.

Type 2 SCD:
A Type 2 SCD supports versioning of dimension members. Often the source system doesn’t store versions, so the data warehouse load process detects and manages changes in a dimension table. In this case, the dimension table must use a surrogate key to provide a unique reference to a version of the dimension member. It also includes columns that define the date range validity of the version (for example, StartDate
and EndDate
) and possibly a flag column (for example, IsCurrent
) to easily filter by current dimension members.
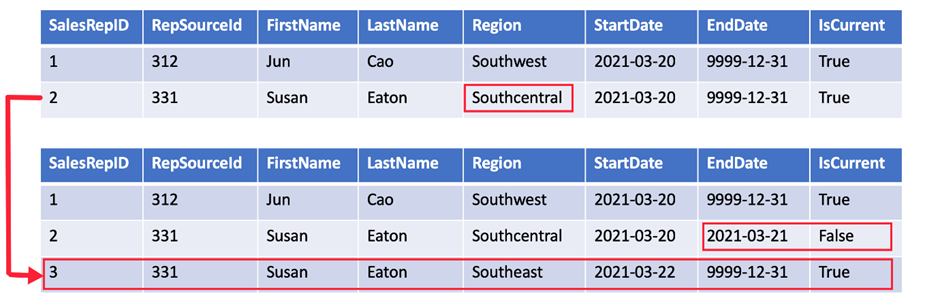
Type 3 SCD:
A Type 3 SCD supports storing two versions of a dimension member as separate columns. The table includes a column for the current value of a member plus either the original or previous value of the member. So Type 3 uses additional columns to track one key instance of history, rather than storing additional rows to track each change like in a Type 2 SCD.
This type of tracking may be used for one or two columns in a dimension table. It is not common to use it for many members of the same table. It is often used in combination with Type 1 or Type 2 members.

Type 6 SCD:
A Type 6 SCD combines Type 1, 2, and 3. When a change happens to a Type 2 member you create a new row with appropriate StartDate and EndDate. In Type 6 design you also store the current value in all versions of that entity so you can easily report on the current value or the historical value.
Using the sales region example, you split the Region column into CurrentRegion
and HistoricalRegion
. The CurrentRegion
always shows the latest value and the HistoricalRegion
shows the region that was valid between the StartDate
and EndDate
. So for the same salesperson, every record would have the latest region populated in CurrentRegion
while HistoricalRegion
works exactly like the region field in the Type 2 SCD example.

60.What is the difference between the Mapping data flow and Wrangling data flow transformation activities in Data Factory?
Mapping data flow activity is a visually designed data transformation activity that allows us to design a graphical data transformation logic without the need to be an expert developer, and executed as an activity within the ADF pipeline on an ADF fully managed scaled-out Spark cluster.
Wrangling data flow activity is a code-free data preparation activity that integrates with Power Query Online in order to make the Power Query M functions available for data wrangling using spark execution.
61.When copying data from or to an Azure SQL Database using Data Factory, what is the firewall option that we should enable to allow the Data Factory to access that database?
Allow Azure services and resources to access this server firewall option.
62. Which activity should you use if you need to use the results from running a query?
A look-up activity can give you results from a query or a program. These results can be a single thing, a list of stuff, or something related to how your program works. You can then use these results in another copy data activity.
63. How are the remaining 90 dataset types in Data Factory used for data access?
You can easily use Azure Synapse Analytics, Azure SQL Database, and certain storage accounts for your data in the mapping data flow feature. It also supports Parquet files from blob storage or Data Lake Storage Gen2. However, for data from other sources, you’ll need to first copy it using the Copy activity before you can work with it in a Data Flow activity.
64. Give more details about the Azure Data Factory’s Get Metadata activity
The Azure Data Factory’s “Get Metadata” activity is a fundamental component of Azure Data Factory (ADF) that allows you to retrieve metadata information about various data-related objects within your data factory or linked services. Metadata includes information about datasets, tables, files, folders, or other data-related entities. Here are some key details about the Get Metadata activity:
Purpose:
- Metadata Retrieval: It is used to retrieve metadata information without moving or processing the actual data. This information can be used for control flow decisions, dynamic parameterization, or for designing complex data workflows.
Use Cases:
- Dynamic Execution: You can use the metadata retrieved by this activity to dynamically control which datasets or files to process or copy in subsequent activities.
- Validation: It can be used to check the existence of datasets or files before attempting to use them, ensuring your pipeline only processes existing resources.
Output:
- The Get Metadata activity outputs the metadata as structured JSON data, which includes details like file names, sizes, column names, table schemas, and more, depending on the metadata type and source.
Dynamic Expressions:
- You can use dynamic expressions within the activity configuration to parameterize your metadata retrieval. For example, you can use expressions to construct folder paths or table names at runtime.
In summary, the Get Metadata activity in Azure Data Factory is a powerful tool for gathering information about your data sources and structures, enabling dynamic and data-driven ETL (Extract, Transform, Load) workflows within your data factory pipelines. It helps you make informed decisions and perform data operations based on the state of your data sources.